Autores
Bedoya, M. (UNIVERSIDAD CATÓLICA DEL MAULE) ; Adasme-carreño, F. (UNIVERSIDAD CATÓLICA DEL MAULE) ; Muñoz-gutierrez, C. (UNIVERSIDAD DE TALCA) ; Hernández-rodríguez, E.W. (UNIVERSIDAD CATÓLICA DEL MAULE) ; Martínez, L. (UNIVERSIDAD DE CAMPINAS) ; Alzate-morales, J. (UNIVERSIDAD DE TALCA)
Resumo
In this work, we propose a novel protocol for conformer generation of small
molecules that uses enhanced sampling
in molecular dynamics (MD) simulations. We termed this new strategy
"Moltiverse", alluding to the universe of
three-dimensional (3D) configurational space of molecules and represents a proof
of concept of the utility of
enhanced sampling for conformer generation. The extended adaptive biasing force
(eABF) algorithm using a single
collective variable of RMSD was employed to explore the conformational space of
the molecules. This first
implementation was benchmarked against the well-established software (RDKit)
showing comparable performance. It is
expected that further optimizations will provide a more comprehensive and
efficient sampling.
Palavras chaves
conformer generation; enhanced sampling; ligands
Introdução
Gaining new knowledge of ligand binding mode in proteins represents an area of
great relevance in the academic
environment and the pharmaceutical industry. However, it is not a simple task.
One way to tackle the problem
using computational methods is to predict the conformations that molecules adopt
when interacting at the
binding site on proteins via molecular docking, free energy methods among
others, on the protein of interest
and thus predict the possible binding mode as well as their possible biological
activity. One crucial initial
step is the generation of ligand conformers to be tested. There are several
freely and commercially available
software for conformer sampling. Some approaches are based on evolutionary
algorithms, geometric searches, as
well as random or systematic generations that are often refined with general
force fields.
On the other hand, enhanced sampling methods rely on MD calculations to explore
the energy landscape
restricted to some collective variables. It is possible to define collective
variables such as the distance,
angles, and dihedral angles between atoms, RMSD, among others, to study the
conformational space of a
molecule. Consequently, it is reasonable to think that these methods could be
used to generate ligand
conformers and even produce ligand conformations similar to protein-bound-like
states. Here we present a first
approach using eABF to generate the conformers of a series of small molecules
with known protein-bound
structures. Initial results show that the strategy can sample numerous
conformations comparable to established
software, many of which are close to bound-state conformations.
Material e métodos
100 ligands were randomly selected from the "Platinum Diverse Dataset"
(FRIEDRICH and MEYDER et al., 2017) which consists of a selection of ligand-
bound
protein structures from the protein data bank (PDB). Initial 3D structures were
generated from the SMILES entries using the RDKit library. The molecules
were prepared for MD simulations with the NAMD (KALÉ et al., 1999) software. The
antechamber (WANG et al., 2006) software was used to generate the ligand’s
parameters and partial charges using the AM1-BCC charge model and the GAFF2
force field (WANG et al., 2004). The structures were energetically minimized
for 100,000 steps in vacuum using the conjugate gradient method. The eABF method
(FU et al., 2016) with a RMSD collective variable as implemented in the
Colvars module (FIORIN et al., 2013) was employed to explore the conformational
space of the molecules. All ligand atoms were included in the collective
RMSD variable. The calculation was divided into 10 windows, and every window
consisted of a width of 0.5 Å of RMSD spanning from 0 to 5 Å. For each window,
a MD simulation was run for 1 ns, and 25 frames equally spaced in time were
stored. Thus, a total of 250 frames of the MD trajectories were considered as
final conformers.
The RDKit (RDKit: Open-source cheminformatics; http://www.rdkit.org) software
was used to also generate 250 conformers per ligand with the standard
geometric distance algorithm in conjunction with the MMFF94 (TOSCO et al., 2014)
force field starting from the same initial 3D structures as before.
The accuracy was measured as the minimum RMSD (Å) between each conformer
generated with RDKit and Moltiverse against the experimentally determined
protein-
bound-like conformation. Non-polar hydrogens were ignored for the RMSD
measurement.
Resultado e discussão
The subset of the chosen 100 molecules has a wide range of numbers of atoms and
rotatable bonds (Figure 1 A,B). The distribution of
rotatable bonds shows a similar trend compared to the original, which is rich in
molecules containing 1 to 6 rotatable bonds(FRIEDRICH and
MEYDER et al., 2017). In general, the greater the number of rotatable bonds, the
greater the degrees of freedom, and the more difficult the
prediction becomes. We have chosen RDKit as reference as it is one of the most
prominent open-source chemoinformatic tools, which contains
several methods, algorithms, and protocols for molecular tasks. RDKit has been
tested against both free access (EBEJER et al., 2012;
FRIEDRICH and MEYDER et al., 2017) and commercial software (FRIEDRICH and DE
BRUYN KOPS et al., 2017) and it has been shown to reproduce
more than 80% of the experimentally-determined conformations with an RMSD below
1.0 Å. Figure 1C shows the distribution of the minimum RMSD
values obtained with the RDKit and Moltiverse approaches.
The range of RMSD values with RDKit (0.02 and 1.50) was slightly lower than the
Moltiverse values (0.05 and 2.06). The cumulative
percentages of RMSD values under different thresholds are shown in Figure 1D.
Below 0.5 Å of RMSD threshold, RDKit predicted experimental
poses in 37% of the ligands while it was 30 % for Moltiverse, but the difference
was larger below 1 Å of RMSD (80 % vs 65 %, respectively).
Below 1.5 Å of RMSD, RDKit predicted 99 % while Moltiverse predicted 90 % of the
ligands. Although RDKit has a better predictive power on
this dataset, both approaches produced conformers within the 2 Å RMSD limit,
which is indicative of good similarity with the experimental
conformations.
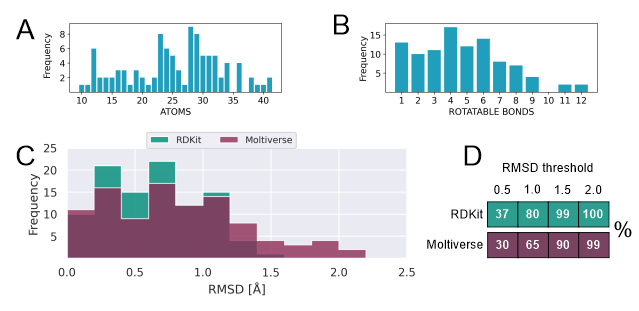
Distribution of the number of atoms (A) and rotatable bonds per molecule (B). C. Minimum RMSD values. D. Percentage of accuracy below RMSD thresholds.
Conclusões
Moltiverse showed comparable albeit slightly worse predictive power than RDKit,
however, the simulation protocol employed here was the simplest approach possible
without post-processing of the MD trajectories. Consequently, it could be argued
that the Moltiverse strategy could be further refined to better explore the
configurational space thus yielding an increased sampling of the ligand
conformation.
Future work will be aimed at refining the collective variables as well as
expanding the testing data to the complete "Platinum diverse dataset".
Agradecimentos
M.B. acknowledges FONDECYT - ANID for his postdoctoral grant Nº 3210774.
This work used resources of the "Centro Nacional de Processamento de Alto
Desempenho em São Paulo (CENAPAD-SP)."
Referências
EBEJER, Jean Paul e MORRIS, Garrett M. e DEANE, Charlotte M. Freely available conformer generation methods: How good are they? Journal of Chemical Information and Modeling, v. 52, n. 5, p. 1146–1158, 25 Maio 2012. Disponível em: <https://pubs.acs.org/doi/abs/10.1021/ci2004658>. Acesso em: 16 set 2022.
FIORIN, Giacomo e KLEIN, Michael L. e HÉNIN, Jérôme. Using collective variables to drive molecular dynamics simulations. Molecular Physics, v. 111, n. 22–23, p. 3345–3362, Dez 2013. Disponível em: <http://www.tandfonline.com/doi/abs/10.1080/00268976.2013.813594>. Acesso em: 18 jul 2019.
FRIEDRICH, Nils Ole e DE BRUYN KOPS, Christina e colab. Benchmarking Commercial Conformer Ensemble Generators. Journal of Chemical Information and Modeling, v. 57, n. 11, p. 2719–2728, 27 Nov 2017. Disponível em: <https://pubs.acs.org/doi/abs/10.1021/acs.jcim.7b00505>. Acesso em: 16 set 2022.
FRIEDRICH, Nils Ole e MEYDER, Agnes e colab. High-Quality Dataset of Protein-Bound Ligand Conformations and Its Application to Benchmarking Conformer Ensemble Generators. Journal of Chemical Information and Modeling, v. 57, n. 3, p. 529–539, 27 Mar 2017. Disponível em: <https://pubs.acs.org/doi/abs/10.1021/acs.jcim.6b00613>. Acesso em: 16 set 2022.
FU, Haohao e colab. Extended Adaptive Biasing Force Algorithm. An On-the-Fly Implementation for Accurate Free-Energy Calculations. Journal of Chemical Theory and Computation, v. 12, n. 8, p. 3506–3513, 9 Ago 2016. Disponível em: <https://pubs.acs.org/doi/abs/10.1021/acs.jctc.6b00447>. Acesso em: 10 set 2020.
KALÉ, Laxmikant e colab. NAMD2: Greater Scalability for Parallel Molecular Dynamics. Journal of Computational Physics, v. 151, n. 1, p. 283–312, Maio 1999. Disponível em: <https://linkinghub.elsevier.com/retrieve/pii/S0021999199962010>.
TOSCO, Paolo e STIEFL, Nikolaus e LANDRUM, Gregory. Bringing the MMFF force field to the RDKit: Implementation and validation. Journal of Cheminformatics, v. 6, n. 1, p. 37, 12 Dez 2014. Disponível em: <https://jcheminf.biomedcentral.com/articles/10.1186/s13321-014-0037-3>. Acesso em: 16 set 2022.
WANG, Junmei e colab. Automatic atom type and bond type perception in molecular mechanical calculations. Journal of Molecular Graphics and Modelling, v. 25, n. 2, p. 247–260, Out 2006. Disponível em: <https://linkinghub.elsevier.com/retrieve/pii/S1093326305001737>. Acesso em: 23 set 2020.
WANG, Junmei e colab. Development and testing of a general amber force field. Journal of computational chemistry, v. 25, n. 9, p. 1157–1174, 15 Jul 2004. Disponível em: <https://pubmed.ncbi.nlm.nih.gov/15116359/>. Acesso em: 16 set 2022.